Product Management Is an AI Candy Store
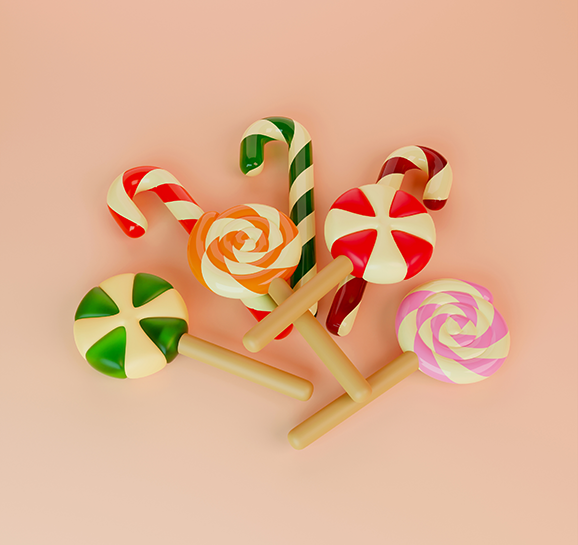
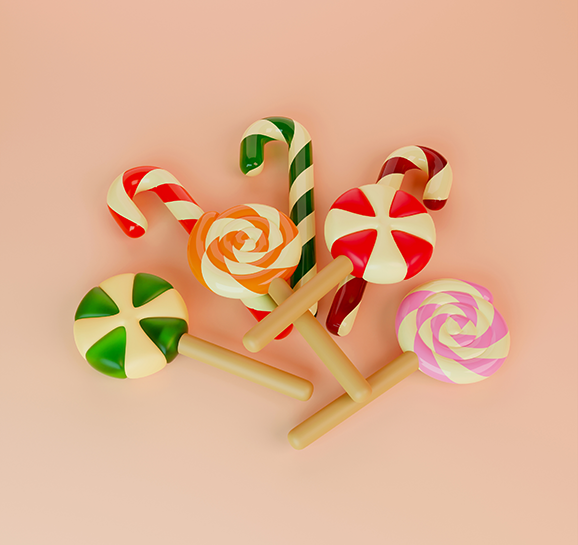
FOR IMMEDIATE RELEASE
Product Management Is an AI Candy Store
Product management is the best function to start your AI transformation.
According to a 2023 study by Accenture, 75% of C-suite executives agree that failing to effectively integrate AI within the next five years could result in business obsolescence. I predict that the 25% of leaders who don’t believe in that future will likely be the first to become obsolete.
AI is poised not just to support decision-making or process data but to lead in driving product innovation and strategic planning. The field of product management is particularly well-suited for AI applications due to its data-rich environment and dynamic nature. AI’s ability to analyze large data sets, identify patterns, predict outcomes, and automate tasks aligns well with the needs of modern product management and strategy.
Here’s why product strategy, management, marketing, and supporting functions are prime candidates for AI integration:
Structured, Repetitive Tasks
Product management and strategy involve numerous repetitive and time-consuming tasks—such as market research, competitor analysis, and design asset development—that AI can automate. This automation enhances efficiency and allows human teams to focus on more strategic and creative aspects of their roles.